Contents
1. Introduction
2. Download and installation
3. XAEM: step by step instruction and explanation
3.1 Preparation for the annotation reference
3.2 Quantification of transcripts
4. A practical copy-paste example of running XAEM
5. Dataset for differential expression (DE) analysis
1. Introduction
This document shows how to use XAEM [Deng et al., 2019] to quantify isoform expression for multiple samples.
What are new in version 0.1.2
- Improve speed and fix bug for building CRP to work with complex annotations such as GENCODE and ENSEMBL, which usually have >200,000 isoforms for hg38. The X-matrix for human Ensembl GRCh38.95 can be downloaded here: X_matrix.RData
What are new in version 0.1.1
- Add standard error for the estimates
- Fix a small bug when separe a CRP into more than 1 CRP due to H_thres
- Fix a small bug in function crpcount() to avoid the error when having only 1 CRP
Older versions
- Code, data and instruction of most XAEM versions are available on the XAEM github site
- Webpage of XAEM version 0.1.1: click here to get there
- Webpage of XAEM version 0.1.0: click here to get there
Software requirements for XAEM:
- R version 3.3.0 or later with installed packages: foreach and doParallel
- C++11 compliant compiler (g++ >= 4.7)
- XAEM is currently tested in Linux OS environment
Annotation reference: XAEM requires a fasta file of transcript sequences and a gtf file of transcript annotation. XAEM supports all kinds of reference and annotation for any species.
The pre-built X-matrix for GRCh38.95 can be downloaded here: X_matrix.RData
In the XAEM paper, we use the UCSC hg19 annotation:
- Download the sequences of transcripts:transcripts.fa.gz
- Download the annotation of transcripts: genes_annotation.gtf.gz
- Download the design matrix X of this annotation: X_matrix.RData (X matrix is an essential object for bias correction and isoform quantification, see Section 4.1.2 for more details)
wget https://www.meb.ki.se/sites/biostatwiki/wp-content/uploads/sites/4/XAEM_datasources/transcripts.fa.gz gunzip transcripts.fa.gz content/uploads/sites/4/XAEM_datasources/genes_annotation.gtf.gz gunzip genes_annotation.gtf.gz wget -O X_matrix.RData https://www.meb.ki.se/sites/biostatwiki/wp-content/uploads/sites/4/2022/09/X_matrix.rdata --no-check-certificate
2. Download and installation
If you use the binary version of XAEM (recommended):
- Download the latest binary version from XAEM website:
wget https://github.com/WenjiangDeng/XAEM/releases/download/v0.1.2/XAEM-binary-0.1.2.tar.gz
- Uncompress to folder
tar -xzvf XAEM-binary-0.1.2.tar.gz
- Move to the XAEM_home directory and do the configuration for XAEM
cd XAEM-binary-0.1.2 bash configure.sh
- Add paths of lib folder and bin folder to LD_LIBRARY_PATH and PATH
export LD_LIBRARY_PATH=/path/to/XAEM-binary-0.1.2/lib:$LD_LIBRARY_PATH export PATH=/path/to/XAEM-binary-0.1.2/bin:$PATH
If you want to build XAEM from sources:
- Download XAEM and move to XAEM_home directory
wget https://github.com/WenjiangDeng/XAEM/releases/download/v0.1.2/XAEM-source-0.1.2.tar.gz tar -xzvf XAEM-source-0.1.2.tar.gz cd XAEM-source-0.1.2 bash configure.sh
- XAEM requires information of flags from Sailfish including DFETCH_BOOST, DBOOST_ROOT, DTBB_INSTALL_DIR and DCMAKE_INSTALL_PREFIX. Please refer to the Sailfish website for more details of these flags.
- Do installation by the following command:
DBOOST_ROOT=/path/to/boostDir/ DTBB_INSTALL_DIR=/path/to/tbbDir/ DCMAKE_INSTALL_PREFIX=/path/to/expectedBuildDir bash install.sh
- After the installation is finished, remember to add the paths of lib folder and bin folder to LD_LIBRARY_PATH and PATH
export LD_LIBRARY_PATH=/path/to/expectedBuildDir/lib:$LD_LIBRARY_PATH export PATH=/path/to/expectedBuildDir/bin:$PATH
Do not forget to replace “/path/to/” by your local path.
3. XAEM: step by step instruction and explanation
XAEM mainly contains the following steps:
- Preparation for the annotation reference: to process the annotation of transcripts to get essential information for transcript quantification. This step includes 1) index transcript sequences and 2) Construct the design matrix X.
- Quantification of transcripts: to get input from multiple RNA-seq samples to do quasi-mapping, generate data for quantifying transcript expression. This step consists of 1) generate equivalence class table; 2) create Y count matrix and 3) estimate transcript expression using AEM algorithm to update the X matrix and transcript (isoform) expression.
3.1 Preparation for the annotation reference
3.1.1 Indexing transcripts
Using TxIndexer to index the transcript sequences in the reference file (transcripts.fa). For example:
wget https://www.meb.ki.se/sites/biostatwiki/wp-content/uploads/sites/4/XAEM_datasources/transcripts.fa.gz gunzip transcripts.fa.gz TxIndexer -t /path/to/transcripts.fa -o /path/to/TxIndexer_idx
3.1.2 Construction of the X matrix (design matrix)
This step constructs the X matrix required by the XAEM pipeline. For users working with human annotation of UCSC hg19 the X matrix can be downloaded here: X_matrix.rdata (need to rename the file to X_matrix.RData).
Given file transcripts.fa containing the transcript sequences of an annotation reference, we construct the design matrix as follows.
- a) Generate simulated RNA-seq data using the R-package “polyester”
## R-packages of "polyester" and "Biostrings" are required Rscript XAEM_home/R/genPolyesterSimulation.R /path/to/transcripts.fa /path/to/design_matrix
- b) Run GenTC to generate Transcript Cluster (TC) using the simulated data. GenTC will generate an eqClass.txt file as the input for next step.
GenTC -i /path/to/TxIndexer_idx -l IU -1 /path/to/design_matrix/sample_01_1.fasta -2 /path/to/design_matrix/sample_01_2.fasta -p 8 -o /path/to/design_matrix
- c) Create a design matrix using buildCRP.R. The parameter setting for this function is as follows.
- in: the input file (eqClass.txt) obtained from the last step.
- out: the output file name (*.RData) which the design matrix will be saved.
- H: (default H=0.025) is the threshold to filter false positive neighbors in each X matrix. (Please refer to the XAEM paper, Section 2.2.1)
Rscript XAEM_home/R/buildCRP.R in=/path/to/design_matrix/eqClass.txt out=/path/to/design_matrix/X_matrix.RData H=0.025
3.2 Quantification of transcripts
Suppose we already created a working directory “XAEM_project” (/path/to/XAEM_project/) for quantification of transcripts.
3.2.1 Generating the equivalence class table
The command to generate equivalence class table for each sample is similar to “sailfish quant”. For example, we want to run XAEM for sample1 and sample2 with 4 cpus:
XAEM -i /path/to/TxIndexer_idx -l IU -1 s1_read1.fasta -2 s1_read2.fasta -p 4 -o /path/to/XAEM_project/sample1 XAEM -i /path/to/TxIndexer_idx -l IU -1 s2_read1.fasta -2 s2_read2.fasta -p 4 -o /path/to/XAEM_project/sample2
- If the data is compressed in gz format. We can combine with gunzip for a decompression on-fly:
XAEM -i /path/to/TxIndexer_idx -l IU -1 <(gunzip -c s1_read1.gz) -2 <(gunzip -c s1_read2.gz) -p 4 -o /path/to/XAEM_project/sample1 XAEM -i /path/to/TxIndexer_idx -l IU -1 <(gunzip -c s2_read1.gz) -2 <(gunzip -c s2_read2.gz) -p 4 -o /path/to/XAEM_project/sample2
3.2.2 Creating Y count matrix
After running XAEM there will be the output of the equivalence class table for multiple samples. We then create the Y count matrix. For example, if we want to run XAEM parallelly using 8 cores, the command is:
Rscript Create_count_matrix.R workdir=/path/to/XAEM_project core=8
3.2.3 Updating the X matrix and transcript expression using AEM algorithm
When finish the construction of Y count matrix, we use the AEM algorithm to update the X matrix. The updated X matrix is then used to estimate the transcript (isoform) expression. The command is as follows.
Rscript AEM_update_X_beta.R workdir=/path/to/XAEM_project core=8 design.matrix=X_matrix.RData isoform.out=XAEM_isoform_expression.RData paralog.out=XAEM_paralog_expression.RData merge.paralogs=FALSE isoform.method=average remove.ycount=TRUE
Parameter setting
- workdir: the path to working directory
- core: the number of cpu cores for parallel computing
- design.matrix: the path to the design matrix
- isoform.out (default=XAEM_isoform_expression.RData): the output contains the estimated expression of individual transcripts, where the paralogs are split into separate isoforms. This file contains two objects: isoform_count and isoform_tpm for estimated counts and normalized values (TPM). The expression of the individual isoforms is calculated with the corresponding setting of parameter “isoform.method” below.
- isoform.method (default=average): to report the expression of the individual members of a paralog as the average or total expression of the paralog set (value=average/total).
- paralog.out (default=XAEM_paralog_expression.RData): the output contains the estimated expression of merged paralogs. This file consists of two objects: XAEM_count and XAEM_tpm for the estimated counts and normalized values (TPM). The standard error of the estimate is supplied in object XAEM_se stored in *.standard_error.RData.
- merge.paralogs (default=TRUE) (*): the parameter to turn on/off (value=TRUE/FALSE) the paralog merging in XAEM. Please see the details of how to use this parameter in the note at the end of this section.
- remove.ycount (default=TRUE): to clean all data of Ycount after use.
The output in this step will be saved in XAEM_isoform_expression.RData, which is the TPM value and raw read counts of multiple samples.
Note: (*) In XAEM pipeline we provide this parameter (merge.paralog) to merge or not merge the paralogs within the updated X matrix (please see XAEM paper Section 2.2.3 and Section 2.3). Turning on (default) the paralog merging step produces a more accurate estimation. Turning off this step can produce the same sets of isoforms between different projects.
4. A practical copy-paste example of running XAEM
This section presents a tutorial to run XAEM pipeline with a toy example. Suppose that input data contain two RNA-seq samples and server supplies 4 CPUs for computation. We can test XAEM by just copy and paste of the example commands.
- Download the binary version of XAEM and do configuration
# Create a working folder mkdir XAEM_example cd XAEM_example # Download the binary version of XAEM wget https://github.com/WenjiangDeng/XAEM/releases/download/v0.1.2/XAEM-binary-0.1.2.tar.gz # Configure the tool tar -xzvf XAEM-binary-0.1.2.tar.gz cd XAEM-binary-0.1.2 bash configure.sh # Add the paths to system export LD_LIBRARY_PATH=$PWD/lib:$LD_LIBRARY_PATH export PATH=$PWD/bin:$PATH cd ..
- Download annotation files and index the transcripts
## download annotation files # Download the design matrix for the human UCSC hg19 annotation wget -O X_matrix.RData https://www.meb.ki.se/sites/biostatwiki/wp-content/uploads/sites/4/2022/09/X_matrix.rdata --no-check-certificate # Download the fasta of transcripts in the human UCSC hg19 annotation wget https://www.meb.ki.se/sites/biostatwiki/wp-content/uploads/sites/4/XAEM_datasources/transcripts.fa.gz gunzip transcripts.fa.gz ## Run XAEM indexer TxIndexer -t transcripts.fa -o TxIndexer_idx
- If using GRCh38.95, download the corresponding annotation files (Homo_sapiens.GRCh38.95.cdna.all.fa and Homo_sapiens.GRCh38.95.gtf) from Ensembl (http://jan2019.archive.ensembl.org/Homo_sapiens/Info/Index) and the X-matrix of GRCh38.95 from here: https://www.dropbox.com/s/x6a693v1y7must0/X_matrix.RData
- Download the RNA-seq data of two samples: sample1 and sample2
## Download input RNA-seq samples # Create a XAEM project to save the data mkdir XAEM_project cd XAEM_project # Download the RNA-seq data wget https://www.meb.ki.se/sites/biostatwiki/wp-content/uploads/sites/4/XAEM_datasources/sample1_read1.fasta.gz wget https://www.meb.ki.se/sites/biostatwiki/wp-content/uploads/sites/4/XAEM_datasources/sample1_read2.fasta.gz wget https://www.meb.ki.se/sites/biostatwiki/wp-content/uploads/sites/4/XAEM_datasources/sample2_read1.fasta.gz wget https://www.meb.ki.se/sites/biostatwiki/wp-content/uploads/sites/4/XAEM_datasources/sample2_read2.fasta.gz cd ..
- Generate the equivalence class tables for these samples
# Number of CPUs CPUNUM=4 # Process for sample 1 XAEM -i TxIndexer_idx -l IU -1 <(gunzip -c XAEM_project/sample1_read1.fasta.gz) -2 <(gunzip -c XAEM_project/sample1_read2.fasta.gz) -p $CPUNUM -o XAEM_project/sample1 # Process for sample 2 XAEM -i TxIndexer_idx -l IU -1 <(gunzip -c XAEM_project/sample2_read1.fasta.gz) -2 <(gunzip -c XAEM_project/sample2_read2.fasta.gz) -p $CPUNUM -o XAEM_project/sample2
- Create Y count matrix
# Note: R packages "foreach" and "doParallel" are required for parallel computing Rscript $PWD/XAEM-binary-0.1.2/R/Create_count_matrix.R workdir=$PWD/XAEM_project core=$CPUNUM design.matrix=$PWD/X_matrix.RData
- Estimate isoform expression using AEM algorithm
Rscript $PWD/XAEM-binary-0.1.2/R/AEM_update_X_beta.R workdir=$PWD/XAEM_project core=$CPUNUM design.matrix=$PWD/X_matrix.RData isoform.out=XAEM_isoform_expression.RData paralog.out=XAEM_paralog_expression.RData
The outputs are stored in the folder of “XAEM_project” including XAEM_isoform_expression.RData and XAEM_paralog_expression.RData.
5. Dataset for differential expression (DE) analysis
In XAEM paper we have used the RNA-seq data from the breast cancer cell line (MDA-MB-231) for DE analysis. Since the original data was generated by our collaborators and not published yet, we provide the equivalence class table by running the read-alignment tool Rapmap, which is the same mapper of Salmon and totally independent from XAEM algorithm. We also prepare the R scripts and the guide to replicate the DE analysis results in the paper.
In this section, we present an instruction to download the data and run the scripts. We try to build the pipeline following the copy-paste manner in shell, but the part of R scripts must be run in R console.
5.1 Download the R-scripts and the design matrix
This step is to download the R-scripts, change directory to the folder containing the R-scripts and download the design matrix.
# Download R-scripts wget https://www.meb.ki.se/sites/biostatwiki/wp-content/uploads/sites/4/XAEM_datasources/brca_singlecell/RDR_brca_singlecell.zip unzip RDR_brca_singlecell.zip cd RDR_brca_singlecell # Download the design matrix wget -O X_matrix.RData https://www.meb.ki.se/sites/biostatwiki/wp-content/uploads/sites/4/2022/09/X_matrix.rdata --no-check-certificate
5.2 Run XAEM from the equivalence class tables which are the output of read-alignment tool Rapmap
Download the data of equivalence classes
# Download the table of equivalence classes of the single cells which are the output of read-alignment tool Rapmap wget https://www.meb.ki.se/sites/biostatwiki/wp-content/uploads/sites/4/XAEM_datasources/brca_singlecell/brca_singlecell_eqclassDir.zip unzip brca_singlecell_eqclassDir.zip
Run XAEM with the input from the equivalence class table using the R-codes below. Note: This step takes about 2 hours using a personal computer with 4 CPUs. Users can consider skipping this step and downloading the available XAEM results for the downstream analysis.
# set the project path projPath=getwd(); setwd(projPath) source("collectDataOfXAEM.R")
If users want to download the available XAEM results
# Download the available results of XAEM wget https://www.meb.ki.se/sites/biostatwiki/wp-content/uploads/sites/4/XAEM_datasources/brca_singlecell/XAEM_results.zip unzip XAEM_results.zip
5.3 Differential-expression analysis of XAEM and other methods
Download the data of cufflinks and salmon. These files contain the read-count data of methods with and without using bias correction.
# Download the results of cufflinks wget https://www.meb.ki.se/sites/biostatwiki/wp-content/uploads/sites/4/XAEM_datasources/brca_singlecell/cufflinks_results.zip unzip cufflinks_results.zip # Download the results of salmons wget https://www.meb.ki.se/sites/biostatwiki/wp-content/uploads/sites/4/XAEM_datasources/brca_singlecell/salmon_results.zip unzip salmon_results.zip
Run the codes below in R to do normalization and differential expression analysis.
# set the project path projPath=getwd(); setwd(projPath) # Normalize the data of three methods XAEM, Salmon and Cufflinks source("Isoform_Expression_CPM_Normalization.R") # Do DE analysis and plot figures source("DEanalysis_plots.R") # output: DE_Analysis.png
The results of the differential expression analysis (Figure 1 below) are the plots (DE_Analysis.png) reproducing Figure 3 of the XAEM paper. Note that due to the randomness of 50 times’ run, the figure might be slightly different from the figure in the paper.
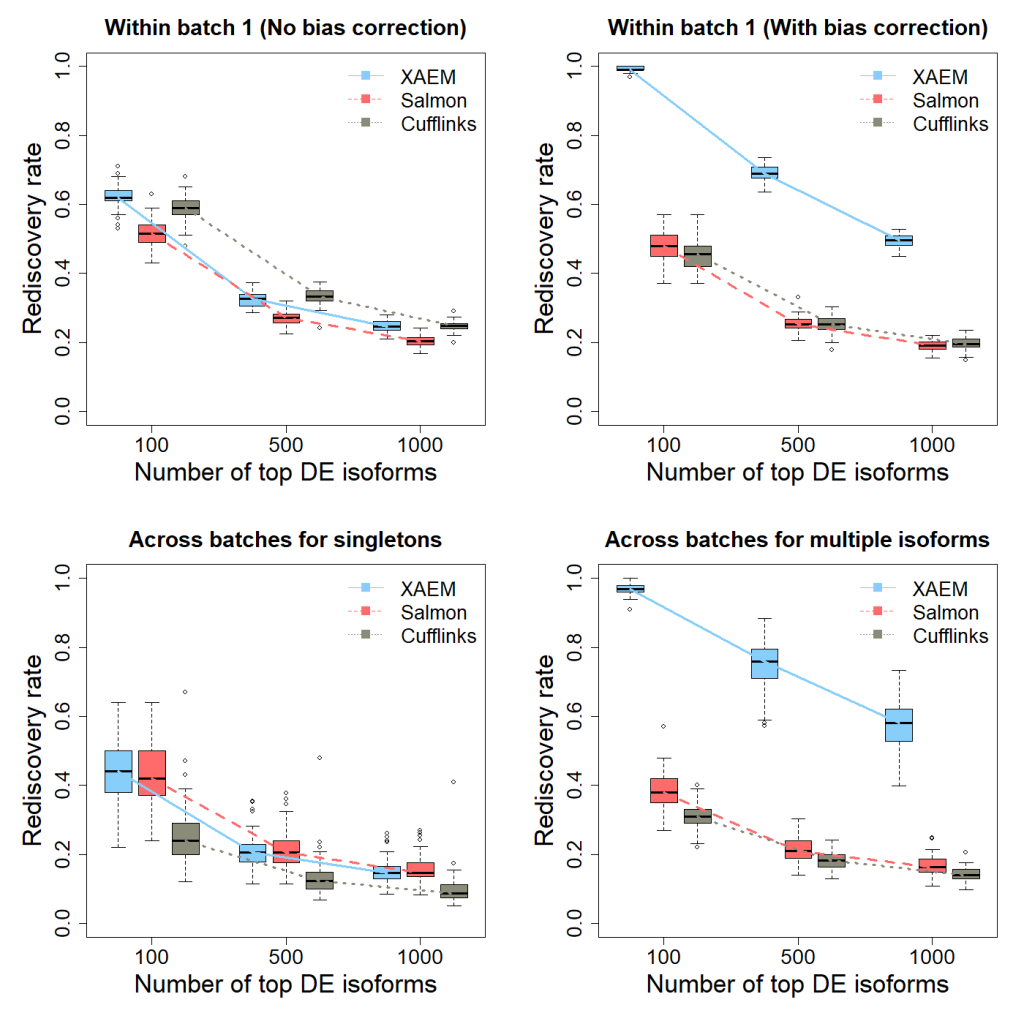
Figure 1. Detection and validation of differentially expressed (DE) isoforms using the MDA- MB-231 scRNA-seq dataset. XAEM, Salmon and Cufflinks are presented in blue-solid, red-dashed and grey-dotted lines, respectively. The x-axis shows the number of top DE isoforms in the training set; the y-axis is the proportion of rediscovery in the validation set. The rediscovery rate (RDR) is calculated by comparing the top 100, 500 and 1000 DE isoforms from the training set with all the significant DE isoforms from the validation set. The boxplots show the RDR from 50 times’ run. (a) Both training set and validation set are constructed using cells from batch 1. The quantification of XAEM, Salmon and Cufflinks is performed without bias correction. (b) The quantification from the three methods are bias- corrected. (c) The training set is constructed using cells from batch 1, while the validation set uses cells from batch 2. The RDR is calculated for only singleton isoforms. (d) The training set is constructed using cells from batch 1, and the validation set using cells from batch 2. The RDR is calculated using only non-paralogs.
References:
- Deng, Wenjiang, Tian Mou, Nifang Niu, Liewei Wang, Yudi Pawitan, and Trung Nghia Vu. 2019. “Alternating EM Algorithm for a Bilinear Model in Isoform Quantification from RNA-Seq Data.” Bioinformatics. https://doi.org/10.1093/bioinformatics/btz640.